Deep Learning recognizes weather and climate patterns
Detecting, classifying and characterizing weather and climate patterns is a fundamental requirement to improve our understanding of extreme events, their formation and how they may change with global warming. These tasks, however, remain challenging across all classes of weather and climate pattern, and especially for extreme events. Deep Learning has revolutionized solutions to pattern recognition and pattern discovery problems resulting in tremendous advances in computer vision, speech recognition, robotics and control systems. We show how deep learning can be similarly impactful in climate science, especially in recognizing and discovering complex weather and climate patterns. We support our claims with results from supervised and semi-supervised learning for classification and detection tasks across a range of weather and climate patterns including tropical cyclones and atmospheric rivers. We present results on state-of-the-art semantic segmentation of multiple classes of event and spatiotemporal tracking of hurricanes. Finally, we present preliminary results on pattern discovery in the context of the Madden Julian Oscillation. We conclude with open grand challenges and opportunities in deep learning for scientific applications.
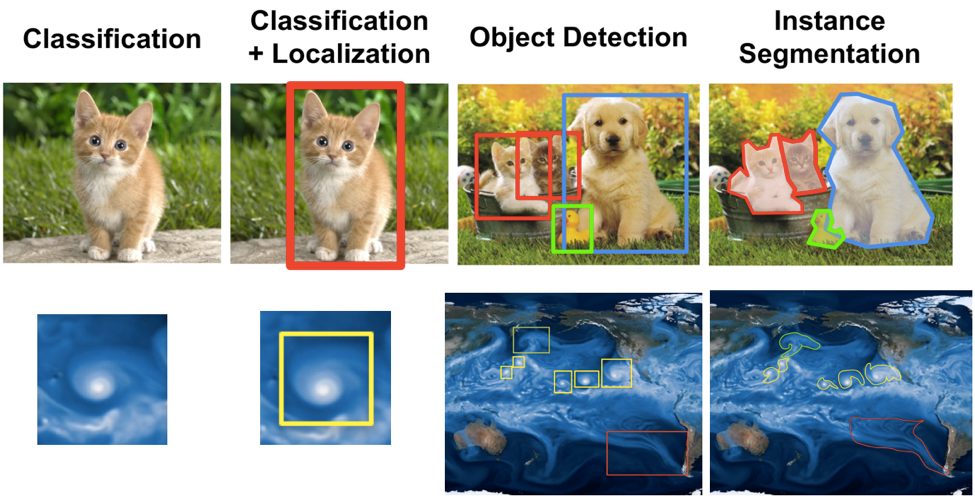