The RUBISCO Role: Analyze, Evaluate, and Diagnose Biogeochemical Models
Complex earth systems prompt complex models, which in turn require complex evaluations
Earth systems―atmosphere, hydrosphere, geosphere, biosphere, and cryosphere―are strongly interconnected through material and energy fluxes, processes, and interactions.
Water and carbon, which are both essential for life on our planet, move through the atmosphere and other systems in cycles (biogeochemical and hydrological cycles respectively), driven by processes that occur at vast scales from the molecular to the planetary, from seconds to eons. All this makes the interactive totality of earth systems large, dynamic, and complex.
Continuous evaluations of numerical simulations of these systems and their future planetary states in earth system models (ESMs) are essential for reducing uncertainties associated with projections of climate change during the remainder of the twentieth century and beyond.
Fidelity Checks
Such evaluations are the aspiration of a science focus area called Reducing Uncertainties in Biogeochemical Interactions through Synthesis and Computation (RUBISCO). Its researchers pay special attention to how biogeochemical processes interact with the water cycle and affect climate.
RUBISCO is sponsored by the Regional and Global Model Analysis (RGMA) program area of the U.S. Department of Energy’s (DOE) Earth and Environmental System Modeling (EESM) Program. Its experts employ the best-available observational data sets to evaluate the fidelity of biogeochemical and hydrological processes in ESMs and increase their predictive accuracy. They also develop open-source benchmarking tools to support model evaluation for DOE’s Energy Exascale Earth System Model (E3SM) and other ESMs.
RUBISCO researchers identify gaps and weaknesses in models, inform new model development, and suggest new measurement and field campaigns.
Here is a glimpse at some recent RUBISCO studies, which hint at the broad scope of their work.
Plant Processes
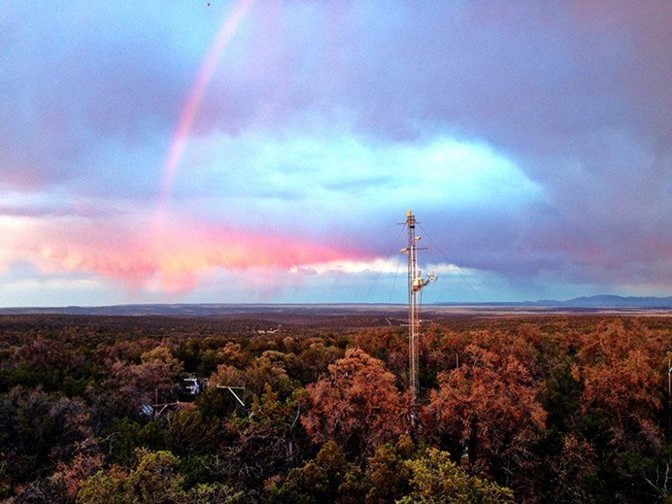
Plant processes strongly affect global terrestrial responses to climate change. A new RUBISCO working group, in collaboration with the AmeriFlux Management Project, focuses on developing, testing, and applying observational methods to improve the performance of land models that account for vegetation and changing climate.
The working group (Hawkins et al., 2020) evaluates ecosystem carbon dioxide (CO2) and water flux data trends in diverse places around the world, studies the effects of extreme events on carbon and water cycling, investigates CO2 sources and sinks, and explores methods for spatial scaling in models―all factors that will impact land-climate interactions in varying ways in the twenty-first century.
Modeling ecosystems requires simulating how carbon is sequestered (“fixed”) on land and how climate change might alter plant and soil processes. Luo and Keenan (2020) provide compelling evidence for photosynthetic light acclimation during long-term CO2 exposure. Liu et al. (2019) show that rising CO2 levels contribute to rising levels of carbon sequestered on land. As for the water cycle, Wu et al. (2020) demonstrate that the models with the most complex representations of plant processes (e.g., evapotranspiration) generate the best estimates of terrestrial evapotranspiration.
And what about grasses? They account for about 30% of net primary productivity in the world, yet their representations in ESMs are oversimplified. Griffith et al. (2020) develop grass functional-trait values to improve land-model representations. Zhu, Zhuang et al. (2019), meanwhile, suggest that even as temperatures continue to rise, tree and grass cover in high northern latitudes may keep soaking up more carbon than released to the atmosphere.
In related work, Xu, Riley et al., (2020) confirm that early leaf growth during spring further warms the climate in cold regions because of its effects on atmospheric circulation and radiative balance. By imposing a 12-day earlier leaf-out in this region, they found 0.3°C–0.7°C surface warming increases in continental arctic warming hotspots.
Deforested land surfaces, increasingly common with climate change, strongly affect surface energy fluxes. Yuan et al. (2021) highlight the need to improve the model predictability of how this happens.
Functional benchmarks from studies like this are helping RUBISCO researchers refine the International Land Model Benchmarking (ILAMB) tool, supported by RGMA.
The ILAMBv2.5 package assesses model fidelity based on 32 variables in five categories from an expanding list of datasets (now more than 50). Designed for modelers and model developers, it is being widely integrated into workflow systems and extended for use in high-latitude and hydrology studies.
ILAMB is expected to be an increasingly powerful platform for identifying model weaknesses.
Soil Carbon Dynamics
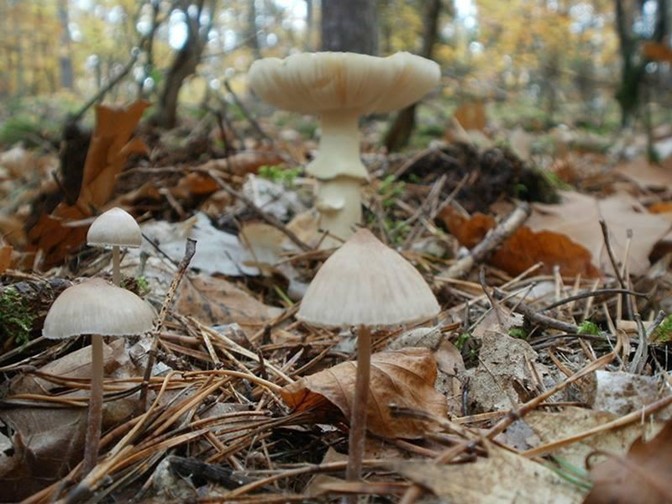
Soils store an enormous amount of carbon. They sequester it from the atmosphere, where its release would accelerate climate warming. Plants and soils remain the primary land-based sources of carbon on timescales shorter than thousands of years.
The turnover time of soil organic carbon (SOC) varies by location, depth below ground, and climate. In a world of increased CO2 emissions, such carbon pools could begin to saturate, further exacerbating warming.
How can we increase carbon storage in major land reservoirs and keep carbon in the ground longer? Adhikari et al. (2020) approached this question by training a machine-learning algorithm to use a large dataset of SOC-related environmental factors (e.g., temperature, soil moisture, and others) and SOC field observations in the continental United States. This study led to a better understanding of how different environmental factors influence the SOC formation at different scales. For example, topography has a larger influence on SOC at finer scales, but at coarser scales climate has a dominant influence.
Other researchers are looking at the age distribution of soil carbon to understand how quickly carbon enters the soil and how long it remains there. Shi et al. (2020) used 789 radiocarbon profiles to create globally gridded datasets of mineral-soil radiocarbon and mean age. The results suggest that over the course of the twenty-first century, soils will accumulate less carbon than current ESMs predict.
Since most ESMs do not track radiocarbon content, Metzler et al. (2020) developed a technique to estimate soil age in model simulations to gauge SOC dynamics in such ESMs. An ongoing source of predictive uncertainty in this regard comes from how land models represent soil carbon dynamics. Tang and Riley (2020) used the results of soil incubation experiments to show that a model of microbe-explicit decomposition processes results in much lower predictive uncertainty than current model structures. These efforts facilitate a more robust assessment of global soil carbon storage dynamics than has been previously possible.
The largest stores of SOC are contained in soils and permafrost at high latitudes and in other cold and water-saturated regions, like mountain ranges and peatlands. Since measurement data from northern circumpolar regions is sparse, Mishra et al. (2020) tested a variety of machine-learning approaches to produce gridded estimates of surface SOC. They found that different methods attributed varying importance to the environmental factors and an ensemble median prediction produces the most realistic spatial estimates of SOC stocks in permafrost regions.
In a larger collaboration, Mishra et al. (2021) recently predicted the spatial variability in SOC stocks at three depth intervals across permafrost-region soils to a depth of 3 meters (9.8 feet). Their analysis produced the first-ever high-resolution map of permafrost-region SOC linked to environmental factors. The map will help researchers evaluate how permafrost soils may respond to changes in climate.
In high latitudes, due to dramatic increases in microbial decomposition, large stores of carbon are the most vulnerable to being released to the atmosphere. Turetsky et al. (2020) developed inventory models to simulate abrupt thaw impacts on the permafrost carbon balance. Their conclusion: models that consider only gradual permafrost thaw substantially underestimate carbon emissions from thawing permafrost.
Decomposition rates increase with warming and may increase terrestrial wetland methane (CH4) emissions. As part of several recent efforts to understand CH4 emissions, RUBISCO scientists participated in the Global Carbon Project methane budget analysis (Saunois et al., 2020). Their findings highlight needed improvements in ESM land model representations of methane production and oxidation (Chang et al., 2021).
Another issue is that fine-scale ecological processes are missing from models, which Keuper et al. (2020) addressed in a recent study. In the rhizosphere priming effect, decomposition rates can accelerate up to fourfold in the presence of plant roots. This mechanism may be especially relevant to thawing permafrost soils since rising temperatures also stimulate plant growth in the Arctic.
The RUBISCO Soil Carbon Dynamics Working Group, formed in 2018 and led by Umakant Mishra at Sandia National Laboratories, is fostering ongoing collaborative studies. As illustrated by studies of Sulman et al. (2020) and Mishra et al. (2021), the Working Group aims to improve model evaluation approaches by combining data synthesis and advanced statistical and machine learning methods.
Biospheric Change in the Arctic
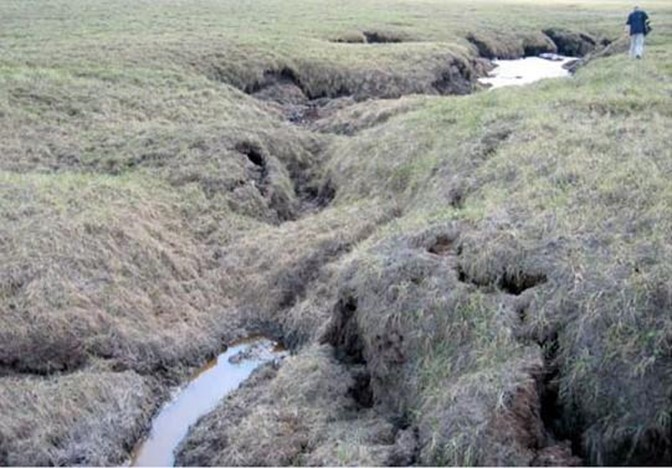
One path of RUBISCO research looks at the marine biosphere of the Arctic, where linkages among hydrology, terrestrial ecosystems, and the marine biosphere are complex and potentially fragile. Some studies show how climate change impacts the hydrological cycle in the far north will have long‐lasting and far‐reaching impacts. Other work explores the potential for ecological tipping points in deep future time.
One of the more robust future projections of climate change is that more rain and snow will fall in northern boreal and Arctic ecosystems. These changes to the hydrological cycle will spur a cascade of ecosystem impacts.
For one, extra warming and rainfall during summer will be accompanied by increases in lightning. Using satellite measurements of lightning strikes and climate model projections, Chen et al. (2021) demonstrate how polar amplification from climate change will almost double the rate of arctic lightning strikes by the end of the century, triggering more fires. The result? An accelerated loss of permafrost carbon stores and forest cover.
In a related analysis, Mekonnen et al. (2019) shows that more fire, in combination with warming, will favor Alaskan deciduous plants, making them dominant in northern ecosystems. In turn, the expansion of deciduous trees and shrubs will affect the carbon cycle, surface energy fluxes, and ecosystem function―altering multiple feedbacks with the climate system.
Using a network of high-latitude soil temperature observations, a mechanistic model, and machine learning, Mekonnen et al. (2021) find that the energy content in precipitation will have an effect on permafrost thaw―one as large as direct effects from warming air. These results challenge current ESM land models, which do not account for precipitation heat content or advective heat transfer in soils.
The precipitation effect comes in addition to concern over rapid permafrost thaw. Turetsky et al. (2020), mentioned previously, shows that accelerated permafrost thaw will lead to even more surface water runoff, erosion, and sediment transport in arctic rivers. Combined, the studies spell dramatic changes coming to coastal zones at high latitudes.
Extra rain in the north is also expected to run across the landscape, dump into rivers, and alter the composition of river discharge into the Arctic Ocean (Jayasinghe et al., 2020). Dissolved organic carbon and other aqueous compounds can attenuate light, produce interfacial films, induce aerosol formation, and release greenhouse gases in high latitude coastal zones and upper ocean waters. The long-term effects of this amplified river flow to the Arctic Ocean are not well understood.
Fu et al. (2020) took the long view, exploring climate change in the north by the year 2300. They found an increased freshwater lens in the Arctic Ocean, which will have a slow, pernicious effect on phytoplankton, therefore inducing reductions in food availability for birds, fish, and whales. By 2300, the flow of organic matter available to support zooplankton, fish, and marine mammals (i.e., “export production”) will decline by more than 50%.
Biosphere-Atmosphere Coupling
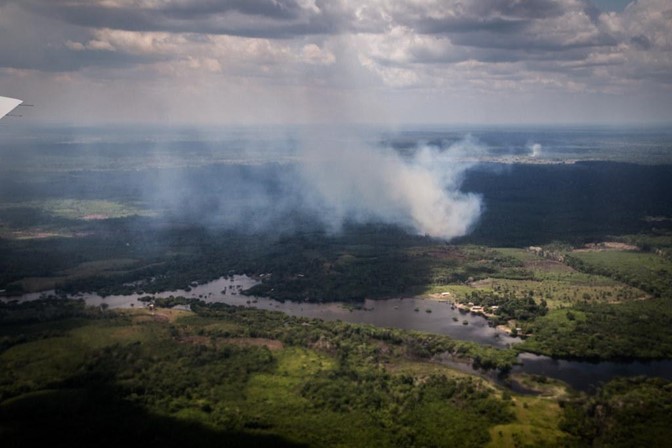
RUBISCO scientists also apply simulations and analyses to interactions between the biosphere and the atmosphere. As climate change takes hold, processes related to soil moisture, fire, plant physiology, and aerosols are likely to be altered.
Biosphere-atmosphere interactions are particularly strong in tropical regions, where large areas of dense forests react to (and alter) atmospheric conditions.
Levine et al. (2019) set out to understand land-atmosphere coupling influences on temperature and the carbon cycle differences between El Niño and La Niña conditions in the Amazon. Working with the DOE’s E3SM, the authors produced three model experiments with 1) full coupling between sea surface and soil, 2) sea surface temperatures (SST) coupling only, and 3) soil moisture coupling only to estimate the contribution of soil moisture variability to the total coupled atmosphere–land response.
These simulations demonstrate that soil moisture variability strengthens and extends temperature and carbon cycle responses to El Niño with wet-season soil moisture anomalies persisting into the dry season in the eastern Amazon. The study demonstrated a unique application of E3SM linked to the tropics version of the Next Generation Ecosystem Experiments at Lawrence Berkeley National Laboratory.
Using both a mesoscale model and ESM, Langenbrunner et al. (2019) find that when less water is transferred to the atmosphere from soil and vegetation in the Amazon, there is an immediate decrease in rainfall. That drastically reduces near-surface moisture and creates a drier, warmer, and deeper boundary layer. The study demonstrates the importance of fast time-scale processes, which can affect stability over a period of hours and in turn influence longer-term vegetation–climate interactions.
Zarakas et al. (2020) quantified plant physiological contributions to the transient climate response (TCR) by using carbon-cycle simulations from the Coupled Model Intercomparison Project (CMIP5 and CMIP6). They found that the physiological effect contributes 0.12°C (or 6.1%) of warming to the TCR, which is defined as a change in global near surface temperature during the 20-yr window centered on the time of atmospheric CO2 doubling relative to preindustrial CO2 concentrations. Plant physiology’s largest contribution to this warming occurs over land, especially in forested regions.
In the Amazon, other scientists wonder, how do fires, tree loss, and atmospheric water dynamics interact? Xu et al. (2020) show that accumulated forest loss since 2000 has warmed and dried the lower atmosphere. Reduced moisture results in larger and more severely dry drought areas, which are vulnerable to more conflagrations and more forest loss in the Amazon’s “arc of deforestation.” Such fires, the authors report, expand through the transition of seasons from wet to dry.
With greenhouse gas emissions still on the rise, there is growing interest in ways to mitigate warming, as well as growing interest in models that predict the effects of such interventions. Most models simulate injecting sulfur dioxide (SO2) into the lower stratosphere―so-called stratospheric aerosol injection. In turn, the models look at predicted changes in air temperature, ocean heat uptake, and atmospheric circulation and precipitation.
A recent RUBISCO study used an ensemble of coupled climate intervention simulations to show that after 2020, terrestrial ecosystems become a stronger carbon sink globally. Yang et al. (2020) estimate that an additional 79 petagrams of carbon (more than 87 billion tons) would be stored on land by the end of this century. The result: as much as a 4% reduction in atmospheric CO2 at 2100 before accounting for ocean feedbacks.
Few models consider ecosystem responses and feedbacks, however―a research gap identified in a cautionary study by Zarnetske et al. (2021). Interventionist strategies to reduce warming, the authors say, first require collaboration between ecologists and climate scientists. Only then can the potential effects on biodiversity and the earth ecosystem be identified.
Toward Better Land and Ocean Models
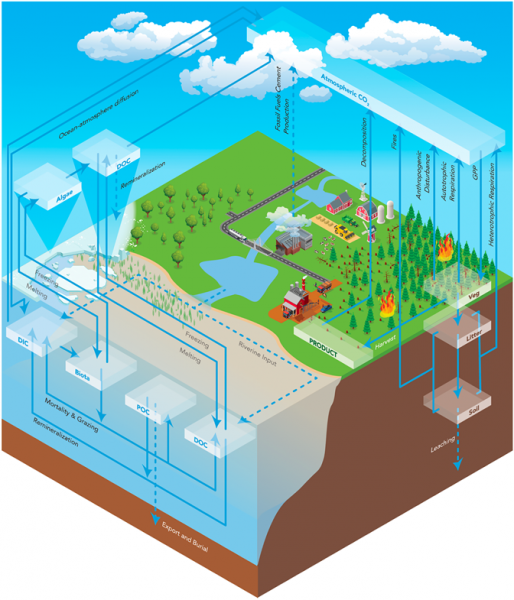
RUBISCO researchers have developed ILAMB (Collier et al., 2018) and the related International Ocean Model Benchmarking (IOMB) package.
ILAMB has been widely adopted at international modeling centers. It was intensively used during the development of the E3SM Land Model version 1 (ELM1) and the Community Land Model version 5 (CLM5).
As a part of joint research with the E3SM project, and as new biogeochemical process representations have been added to the land model, Zhu, Riley et al. (2019) assisted in evaluating ELM1. Once the land model was ready, and once modelers performed fully coupled E3SM simulations, RUBISCO participated in evaluating those simulations and comparing them to a prior generation of ESMs (Burrows et al., 2020).
Similarly, ILAMB played an important role in understanding changes incorporated into the CLM5 model (Lawrence et al., 2019; Bonan et al., 2019). A variety of ongoing development efforts in ILAMB are directed at making the package more useful in understanding why models exhibit certain biases.
These efforts include new work to incorporate different kinds of observational uncertainty into key datasets. That includes the best ways to bring in data from experiments (Wieder et al., 2019) and the most flexible ways to display results in a web-based dashboard. Such a dashboard can integrate model evaluation output from a variety of similar packages.
Both ILAMB and its ocean analog, IOMB, are useful in understanding the range of projections in model intercomparison projects. ILAMB is used routinely by the Global Carbon Project in its annual Global Carbon Budget to evaluate the terrestrial biosphere models that contribute to budget estimates (Friedlingstein et al., 2020, 2019; Le Quéré et al., 2018).
ILAMB and IOMB were used to evaluate two generations of land and ocean components of earth system models for the Intergovernmental Panel on Climate Change Sixth Assessment Report (AR6) from Working Group I, released in August 2021. That effort culminated in a large figure (Figure 5.22) in the AR6 in Chapter 5 on Global Carbon and Other Biogeochemical Cycles and Feedbacks.
RUBISCO References
Plant Processes
- Griffith, D. M., C. P. Osborne, E. J. Edwards, S. Bachle, D. J. Beerling, W. J. Bond, T. J. Gallaher, B. R. Helliker, C. E. R. Lehmann, L. Leatherman, J. B. Nippert, S. Pau, F. Qiu, William J. Riley, M. D. Smith, C. A. E. Strömberg, L. Taylor, M. Ungerer, and C. J. Still (2020), Lineage-based Functional Types: Characterising Functional Diversity to Enhance the Representation of Ecological Behaviour in Land Surface Models, New Phytol., 228(1):15–23, doi:10.1111/nph.16773.
- Hawkins, L. R., Jitendra Kumar, Xiangzhong Luo, D. Sihi, and S. Zhou (2020), Measuring, Monitoring, and Modeling Ecosystem Cycling, Eos Trans. AGU, 101, doi:10.1029/2020EO147717.
- Liu, Y., S. Piao, T. Gasser, P. Ciais, H. Yang, H. Wang, Trevor F. Keenan, M. Huang, S. Wan, J. Song, K. Wang, I. A. Janssens, J. Peñuelas, C. Huntingford, X. Wang, M. Altaf Arain, Y. Fang, J. B. Fisher, M. Huang, D. N. Huntzinger, A. Ito, A. K. Jain, Jiafu Mao, A. M. Michalak, C. Peng, B. Poulter, C. Schwalm, Xiaoying Shi, H. Tian, Y. Wei, N. Zeng, Q. Zhu, and T. Wang (2019), Field-experiment Constraints on the Enhancement of the Terrestrial Carbon Sink by CO2 Fertilization, Nature Geosci., 12(10):809–814, doi:10.1038/s41561-019-0436-1.
- Luo, Xiangzhong, and Trevor F. Keenan (2020), Global Evidence for the Acclimation of Ecosystem Photosynthesis to Light, Nat. Ecol. Evol., 4(10):1351–1357, doi:10.1038/s41559-020-1258-7.
- Wu, G., Xitian Cai, Trevor F. Keenan, S. Li, Xiangzhong Luo, J. B. Fisher, R. Cao, F. Li, A. J. Purdy, W. Zhao, X. Sun, and Z. Hu (2020), Evaluating Three Evapotranspiration Estimates from Model of Different Complexity over China Using the ILAMB Benchmarking System, J. Hydrol., 590, 125553, doi:10.1016/j.jhydrol.2020.125553.
- Xu, Xiyan, William J. Riley, Charles D. Koven, G. Jia, and X. Zhang (2020), Earlier Leaf-out Warms Air in the North, Nat. Clim. Change, 10(4):370–375, doi:10.1038/s41558-020-0713-4.
- Yuan, K., Qing Zhu, S. Zheng, L. Zhao, M. Chen, William J. Riley, Xitian Cai, H. Ma, F. Li, H. Wu, and L. Chen (2021), Deforestation Reshapes Land-surface Energy-flux Partitioning, Environ. Res. Lett., 16(2), 024014, doi:10.1088/1748-9326/abd8f9.
- Zhu, P., Q. Zhuang, L. Welp, P. Ciais, M. Heimann, B. Peng, W. Li, C. Bernacchi, C. Roedenbeck, and Trevor F. Keenan (2019), Recent Warming Has Resulted in Smaller Gains in Net Carbon Uptake in Northern High Latitudes, J. Clim., 32(18):5849–5863, doi:10.1175/JCLI-D-18-0653.1.
Soil Carbon Dynamics
- Adhikari, K., Umakant Mishra, P. R. Owens, Z. Libohova, S. A. Wills, William J. Riley, Forrest M. Hoffman, and D. R. Smith (2020), Importance and Strength of Environmental Controllers of Soil Organic Carbon Changes with Scale, Geoderma, 375, 114472, doi:10.1016/j.geoderma.2020.114472.
- Chang, Kuang-Yu, William J. Riley, S. H. Knox, R. B. Jackson, G. McNicol, B. Poulter, M. Aurela, D. Baldocchi, S. Bansal, G. Bohrer, D. I. Campbell, A. Cescatti, H. Chu, K. B. Delwiche, A. Desai, E. Euskirchen, T. Friborg, M. Goeckede, G. Holm, M. Kang, Trevor F. Keenan, K. W. Krauss, A. Lohila, I. Mammarella, A. Miyata, M. B. Nilsson, A. Noormets, D. Papale, B. R. K. Runkle, Y. Ryu, T. Sachs, K. V. R. Schäfer, H. P. Schmid, N. Shurpali, O. Sonnentag, A. C. I. Tang, M. S. Torn, C. Trotta, M. Ueyama, R. Vargas, T. Vesala, L. Windham-Myers, Z. Zhang, and D. Zona (2021), Global Wetland Methane Emissions Have Hysteretic Responses to Seasonal Temperature, Nature Commun., 12(1), 2266, doi:10.1038/s41467-021-22452-1.
- Keuper, F., B. Wild, M. Kummu, C. Beer, G. Blume-Werry, S. Fontaine, K. Gavazov, N. Gentsch, G. Guggenberger, G. Hugelius, M. Jalava, Charles D. Koven, E. J. Krab, P. Kuhry, S. Monteux, A. Richter, T. Shahzad, J. T. Weedon, and E. Dorrepaal (2020), Carbon Loss from Northern Circumpolar Permafrost Soils Amplified by Rhizosphere Priming, Nature Geosci., 13(8):560–565, doi:10.1038/s41561-020-0607-0.
- Metzler, H., Qing Zhu, William J. Riley, A. Hoyt, M. Müller, and C. A. Sierra (2020), Mathematical Reconstruction of Land Carbon Models From Their Numerical Output: Computing Soil Radiocarbon From 12C Dynamics, J. Adv. Model. Earth Sy., 12(1), e2019MS001776, doi:10.1029/2019MS001776.
- Mishra, Umakant, G. Hugelius, E. Shelef, Y. Yang, J. Strauss, A. Lupachev, J. W. Harden, J. D. Jastrow, C.-L. Ping, William J. Riley, E. A. G. Schuur, R. Matamala, M. Siewert, L. E. Nave, Charles D. Koven, M. Fuchs, J. Palmtag, P. Kuhry, C. C. Treat, S. Zubrzycki, Forrest M. Hoffman, B. Elberling, P. Camill, A. Veremeeva, and A. Orr (2021), Spatial Heterogeneity and Environmental Predictors of Permafrost Region Soil Organic Carbon Stocks, Sci. Adv., doi:10.1126/sciadv.aaz5236.
- Mishra, Umakant, Sagar Gautam, William J. Riley, and Forrest M. Hoffman (2020), Ensemble Machine Learning Approach Improves Predicted Spatial Variation of Surface Soil Organic Carbon Stocks in Data-Limited Northern Circumpolar Region, Front. Big Data, 3:40, doi:10.3389/fdata.2020.528441.
- Saunois, M., A. R. Stavert, B. Poulter, P. Bousquet, J. G. Canadell, R. B. Jackson, P. A. Raymond, E. J. Dlugokencky, S. Houweling, P. K. Patra, P. Ciais, V. K. Arora, D. Bastviken, P. Bergamaschi, D. R. Blake, G. Brailsford, L. Bruhwiler, K. M. Carlson, M. Carrol, S. Castaldi, N. Chandra, C. Crevoisier, P. M. Crill, K. Covey, C. L. Curry, G. Etiope, C. Frankenberg, N. Gedney, M. I. Hegglin, L. Höglund-Isaksson, G. Hugelius, M. Ishizawa, A. Ito, G. Janssens-Maenhout, K. M. Jensen, F. Joos, T. Kleinen, P. B. Krummel, R. L. Langenfelds, G. G. Laruelle, L. Liu, T. Machida, S. Maksyutov, K. C. McDonald, J. McNorton, P. A. Miller, J. R. Melton, I. Morino, J. Müller, F. Murguia-Flores, V. Naik, Y. Niwa, S. Noce, S. O’Doherty, R. Parker, C. Peng, S. Peng, G. P. Peters, C. Prigent, R. Prinn, M. Ramonet, P. Regnier, William J. Riley, J. A. Rosentreter, A. Segers, I. J. Simpson, H. Shi, S. J. Smith, L. P. Steele, B. F. Thornton, H. Tian, Y. Tohjima, F. N. Tubiello, A. Tsuruta, N. Viovy, A. Voulgarakis, T. S. Weber, M. van Weele, G. R. van der Wer, R. F. Weiss, D. Worthy, D. Wunch, Y. Yin, Y. Yoshida, W. Zhang, Z. Zhang, Y. Zhao, B. Zheng, Qing Zhu, Q. Zhu, and Q. Zhuang (2020), The Global Methane Budget 2000–2017, Earth Syst. Sci. Data, 12(3), 1561–1623, doi:10.5194/essd-12-1561-2020.
- Shi, Zheng, S. D. Allison, Y. He, Paul A. Levine, A. M. Hoyt, J. Beem-Miller, Qing Zhu, W. R. Wieder, S. E. Trumbore, and James T. Randerson (2020), The age distribution of global soil carbon inferred from radiocarbon measurements, Nature Geosci., 13(8):555–559, doi:10.1038/s41561-020-0596-z.
- Soong, J. L., C. L. Phillips, C. Ledna, Charles D. Koven, and M. S. Torn (2020), CMIP5 Models Predict Rapid and Deep Soil Warming Over the 21st Century, J. Geophys. Res. Biogeosci., 125(2), e2019JG005266, doi:10.1029/2019JG005266.
- Sulman, B. N., J. Harden, Y. He, C. Treat, Charles D. Koven, Umakant Mishra, J. A. O'Donnell, and L. E. Nave (2020), Land Use and Land Cover Affect the Depth Distribution of Soil Carbon: Insights From a Large Database of Soil Profiles, Front. Environ. Sci., 8:146, doi:10.3389/fenvs.2020.00146.
- Tang, Jinyun, and William J. Riley (2020), Linear Two-pool Models Are Insufficient to Infer Soil Organic Matter Decomposition Temperature Sensitivity from Incubations, Biogeochemistry, doi:10.1007/s10533-020-00678-3.
- Turetsky, M. R., B. W. Abbott, M. C. Jones, K. W. Anthony, D. Olefeldt, E. A. G. Schuur, G. Grosse, P. Kuhry, G. Hugelius, Charles D. Koven, David M. Lawrence, C. Gibson, A. B. K. Sannel, and A. D. McGuire (2020), Carbon Release Through Abrupt Permafrost Thaw, Nature Geosci., 13(2):138–143, doi:10.1038/s41561-019-0526-0.
Biospheric Change in the Arctic
- Chen, Yang, D. M. Romps, J. T. Seeley, S. Veraverbeke, William J. Riley, Zelalem Mekonnen, and James T. Randerson (2021), Future Lightning Increases in the Arctic: Implications for Fire and Permafrost Carbon, Nat. Clim. Change, doi:10.1038/s41558-021-01011-y in press.
- Fu, Weiwei, J. Keith Moore, F. W. Primeau, K. Lindsay, and James T. Randerson (2020), A Growing Freshwater Lens in the Arctic Ocean with Sustained Climate Warming Disrupts Marine Ecosystem Function, Geophys. Res. Lett., 125(12), e2020JG005693, doi:10.1029/2020JG005693.
- Jayasinghe, Amadini, Scott M. Elliott, A. Piliouras, J. Clement Kinney, G. Gibson, N. Jeffery, Forrest M. Hoffman, Jitendra Kumar, and O. Wingenter (2020), Modeling Functional Organic Chemistry in Arctic Rivers: An Idealized Siberian System, Atmos., 11(10), 1090, doi:10.3390/atmos11101090.
- Mekonnen, Zelalem A., William J. Riley, James T. Randerson, R. F. Grant, and B. M. Rogers (2019), Expansion of High-latitude Deciduous Forests Driven by Interactions between Climate Warming and Fire, Nature Plants, 5(9):952–958, doi:10.1038/s41477-019-0495-8.
- Mekonnen, Zelalem A., William J. Riley, R. F. Grant, and V. E. Romanovsky (2021), Changes in Precipitation and Air Temperature Contribute Comparably to Permafrost Degradation in a Warmer Climate, Environ. Res. Lett., 16(2), 024008, doi:10.1088/1748-9326/abc444.
Biosphere-Atmosphere Coupling
- Langenbrunner, Baird, M. S. Pritchard, G. J. Kooperman and James T. Randerson (2019), Why Does Amazon Precipitation Decrease When Tropical Forests Respond to Increasing CO2?, Earth’s Future, 7(4):450–468, doi:10.1029/2018EF001026.
- Levine, Paul A., James T. Randerson, Yang Chen, M. S. Pritchard, Min Xu, and Forrest M. Hoffman (2019), Soil Moisture Variability Intensifies and Prolongs Eastern Amazon Temperature and Carbon Cycle Response to El Niño-Southern Oscillation, J. Clim., 32(4):1273–1292, doi:10.1175/JCLI-D-18-0150.1.
- Xu, Xiyan, G. Jia, X. Zhang, William J. Riley, and Y. Xue (2020), Climate Regime Shift and Forest Loss Amplify Fire in Amazonian Forests, Glob. Change Biol., 26(10):5874–5885, doi:10.1111/gcb.15279.
- Yang, Cheng-En, Forrest M. Hoffman, D. M. Ricciuto, S. Tilmes, L. Xia, D. G. MacMartin, B. Kravitz, J. H. Richter, M. Mills, and J. S. Fu (2020), Assessing Terrestrial Biogeochemical Feedbacks in a Strategically Geoengineered Climate, Environ. Res. Lett., 15(10), 104043, doi:10.1088/1748-9326/abacf7.
- Zarakas, C. M., A. L. S. Swann, M. M. Laguë, K. C. Armour, and James T. Randerson (2020), Plant Physiology Increases the Magnitude and Spread of the Transient Climate Response to CO2 in CMIP6 Earth System Models, J. Clim., 33(19):8561–8578, doi:10.1175/JCLI-D-20-0078.1.
- Zarnetske, P. L., J. Gurevitch, J. Franklin, P. Groffman, C. Harrison, J. Hellmann, Forrest M. Hoffman, S. Kothari, A. Robock, S. Tilmes, D. Visioni, J. Wu, L. Xia, and Cheng-En Yang (2021), Potential Ecological Impacts of Climate Intervention by Reflecting Sunlight to Cool Earth, Proceedings of the National Academy of Sciences 118(15),: e1921854118, doi:10.1073/pnas.1921854118.
A Better Land Model
- Bonan, G. B., D. L. Lombardozzi, W. R. Wieder, K. W. Oleson, David M. Lawrence, Forrest M. Hoffman, and Nathan Collier (2019), Model Structure and Climate Data Uncertainty in Historical Simulations of the Terrestrial Carbon Cycle (1850–2014), Global Biogeochem. Cycles, 33(10):1310–1326, doi:10.1029/2019GB006175.
- Burrows, S. M., M. Maltrud, X. Yang, Qing Zhu, N. Jeffery, Xiaoying Shi, D. M. Ricciuto, S. Wang, G. Bisht, Jinyun Tang, J. Wolfe, B. E. Harrop, B. Singh, L. Brent, S. Baldwin, T. Zhou, P. Cameron-Smith, N. Keen, Nathan Collier, Min Xu, E. C. Hunke, Scott M. Elliott, A. K. Turner, H.-Y. Li, H. Wang, J.-C. Golaz, B. Bond-Lamberty, Forrest M. Hoffman, William J. Riley, P. E. Thornton, K. Calvin, and L. R. Leung (2020), The DOE E3SM v1.1 Biogeochemistry Configuration: Description and Simulated Ecosystem-climate Responses to Historical Changes in Forcing, J. Adv. Model. Earth Syst., 12(9), e2019MS001766, doi:10.1029/2019MS001766.
- Collier, Nathan, Forrest M. Hoffman, David M. Lawrence, Gretchen Keppel-Aleks, Charles D. Koven, William J. Riley, Mingquan Mu, and James T. Randerson (2018), The International Land Model Benchmarking (ILAMB) System: Design, Theory, and Implementation, J. Adv. Model. Earth Syst., 10(11):2731–2754, doi:10.1029/2018MS001354.
- Friedlingstein, P., M. W. Jones, M. O'Sullivan, R. M. Andrew, J. Hauck, G. P. Peters, W. Peters, J. Pongratz, S. Sitch, C. Le Quéré, D. C. E. Bakker, J. G. Canadell, P. Ciais, R. B. Jackson, P. Anthoni, L. Barbero, A. Bastos, V. Bastrikov, M. Becker, L. Bopp, E. Buitenhuis, N. Chandra, F. Chevallier, L. P. Chini, K. I. Currie, R. A. Feely, M. Gehlen, D. Gilfillan, T. Gkritzalis, D. S. Goll, N. Gruber, S. Gutekunst, I. Harris, V. Haverd, R. A. Houghton, G. Hurtt, T. Ilyina, A. K. Jain, E. Joetzjer, J. O. Kaplan, E. Kato, K. Klein Goldewijk, J. I. Korsbakken, P. Landschützer, S. K. Lauvset, N. Lefèvre, A. Lenton, S. Lienert, D. Lombardozzi, G. Marland, P. C. McGuire, J. R. Melton, N. Metzl, D. R. Munro, J. E. M. S. Nabel, S.-I. Nakaoka, C. Neill, A. M. Omar, T. Ono, A. Peregon, D. Pierrot, B. Poulter, G. Rehder, L. Resplandy, E. Robertson, C. Rödenbeck, R. Séférian, J. Schwinger, N. Smith, P. P. Tans, H. Tian, B. Tilbrook, F. N. Tubiello, G. R. van der Werf, A. J. Wiltshire, and S. Zaehle (2019), Global Carbon Budget 2019, Earth Syst. Sci. Data, 11:1783–1838, doi:10.5194/essd-11-1783-2019.
- Friedlingstein, P., M. O'Sullivan, M. W. Jones, R. M. Andrew, J. Hauck, A. Olsen, G. P. Peters, W. Peters, J. Pongratz, S. Sitch, C. Le Quéré, J. G. Canadell, P. Ciais, R. B. Jackson, S. Alin, L. E. O. C. Aragão, A. Arneth, V. Arora, N. R. Bates, M. Becker, A. Benoit-Cattin, H. C. Bittig, L. Bopp, S. Bultan, N. Chandra, F. Chevallier, L. P. Chini, W. Evans, L. Florentie, P. M. Forster, T. Gasser, M. Gehlen, D. Gilfillan, T. Gkritzalis, L. Gregor, N. Gruber, I. Harris, K. Hartung, V. Haverd, R. A. Houghton, T. Ilyina, A. K. Jain, E. Joetzjer, K. Kadono, E. Kato, V. Kitidis, J. I. Korsbakken, P. Landschützer, N. Lefèvre, A. Lenton, S. Lienert, Z. Liu, D. Lombardozzi, G. Marland, N. Metzl, D. R. Munro, J. E. M. S. Nabel, S.-I. Nakaoka, Y. Niwa, K. O'Brien, T. Ono, P. I. Palmer, D. Pierrot, B. Poulter, L. Resplandy, E. Robertson, C. Rödenbeck, J. Schwinger, R. Séférian, I. Skjelvan, A. J. P. Smith, A. J. Sutton, T. Tanhua, P. P. Tans, H. Tian, B. Tilbrook, G. van der Werf, N. Vuichard, A. P. Walker, R. Wanninkhof, A. J. Watson, D. Willis, A. J. Wiltshire, W. Yuan, X. Yue, and S. Zaehle (2020), Global Carbon Budget 2020, Earth Syst. Sci. Data, 12:3269–3340, doi:10.5194/essd-12-3269-2020.
- Lawrence, David M., R. A. Fisher, Charles D. Koven, K. W. Oleson, S. C. Swenson, G. B. Bonan, Nathan Collier, B. Ghimire, L. van Kampenhout, D. Kennedy, E. Kluzek, P. J. Lawrence, F. Li, H. Li, D. Lombardozzi, William J. Riley, W. J. Sacks, M. Shi, M. Vertenstein, W. R. Wieder, C. Xu, A. A. Ali, A. M. Badger, G. Bisht, M. van den Broeke, M. A. Brunke, S. P. Burns, J. Buzan, M. Clark, A. Craig, K. Dahlin, B. Drewniak, J. B. Fisher, M. Flanner, A. M. Fox, P. Gentine, Forrest M. Hoffman, Gretchen Keppel-Aleks, R. Knox, S. Kumar, J. Lenaerts, L. R. Leung, W. H. Lipscomb, Y. Lu, J. D. Pelletier, J. Perket, James T. Randerson, D. M. Ricciuto, B. M. Sanderson, A. Slater, Z. M. Subin, Jinyun Tang, R. Q. Thomas, M. V. Martin, X. Zeng (2019), The Community Land Model Version 5: Description of New Features, Benchmarking, and Impact of Forcing Uncertainty, J. Adv. Model. Earth Syst., 11(12):4245–4287, doi:10.1029/2018MS001583.
- Le Quéré, C., R. M. Andrew, P. Friedlingstein, S. Sitch, J. Hauck, J. Pongratz, P. A. Pickers, J. I. Korsbakken, G. P. Peters, J. G. Canadell, A. Arneth, V. K. Arora, L. Barbero, A. Bastos, L. Bopp, F. Chevallier, L. P. Chini, P. Ciais, S. C. Doney, T. Gkritzalis, D. S. Goll, I. Harris, V. Haverd, Forrest M. Hoffman, M. Hoppema, R. A. Houghton, G. Hurtt, T. Ilyina, A. K. Jain, T. Johannessen, C. D. Jones, E. Kato, R. F. Keeling, K. K. Goldewijk, P. Landschützer, N. Lefèvre, S. Lienert, Z. Liu, D. Lombardozzi, N. Metzl, D. R. Munro, J. E. M. S. Nabel, S.-I. Nakaoka, C. Neill, A. Olsen, T. Ono, P. Patra, A. Peregon, W. Peters, P. Peylin, B. Pfeil, D. Pierrot, B. Poulter, G. Rehder, L. Resplandy, E. Robertson, M. Rocher, C. Rödenbeck, U. Schuster, J. Schwinger, R. Séférian, I. Skjelvan, T. Steinhoff, A. Sutton, P. P. Tans, H. Tian, B. Tilbrook, F. N. Tubiello, I. T. van der Laan-Luijkx, G. R. van der Werf, N. Viovy, A. P. Walker, A. J. Wiltshire, R. Wright, S. Zaehle, and B. Zheng (2018), Global Carbon Budget 2018, Earth Syst. Sci. Data, 10(4):2141–2194, doi:10.5194/essd-10-2141-2018.
- Wieder, W. R., David M. Lawrence, R. A. Fisher, G. B. Bonan, S. J. Cheng, C. L. Goodale, A. S. Grandy, Charles D. Koven, D. L. Lombardozzi, K. W. Oleson, and R. Q. Thomas 2019, Beyond Static Benchmarking: Using Experimental Manipulations to Evaluate Land Model Assumptions, Global Biogeochem. Cycles, 33(10):1289–1309, doi:10.1029/2018GB006141.
- Zhu, Qing, William J. Riley, Jinyun Tang, Nathan Collier, Forrest M. Hoffman, Xiaojuan Yang, and Gautam Bisht (2019), Representing Nitrogen, Phosphorus, and Carbon Interactions in the E3SM Land Model: Development and Global Benchmarking, J. Adv. Model. Earth Syst., 11(7):2238–2258, doi:10.1029/2018MS001571.