Advanced Techniques Help Decipher Past Climate Change
PCMDI Contributes to Successful ForceSMIP Hackathon
Historical changes in climate are principally driven by anthropogenic external forcings, such as aerosol and greenhouse gas emissions, and natural forcings, such as volcanic eruptions. Natural internal variability is superimposed on these “forced” changes in climate and can amplify or suppress regional and global climate trends. Contributions from unforced, internal variations in climate can complicate our interpretation of historical climate change, undermining the ability of climate scientists to predict future change.
The Forced Component Estimation Statistical Method Intercomparison Project (ForceSMIP) is intended to address this problem by coordinating efforts and evaluating techniques to quantify forced-versus-unforced changes in climate. Given the increasing availability of large ensembles of model simulations and the successful application of novel artificial intelligence (AI) and machine learning (ML) techniques, a key question is whether new methods can improve existing techniques to disentangle forced-versus-unforced climate variability and change.
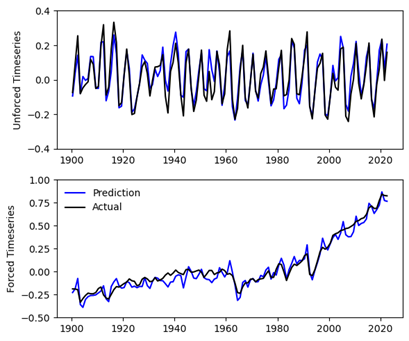
Participants in this project will develop novel statistical and machine learning methods to estimate the spatio-temporally resolved forced climate response across a range of geophysical fields, including surface and atmospheric temperature, surface pressure, and precipitation. Participants will use the known forced climate response derived from single model initial-condition large ensembles to train and validate their methods.
They will then attempt to estimate the forced response in individual time series of out-of-sample data in which the true forced climate response is only known to organizers of ForceSMIP, who will evaluate the accuracy of submitted methods. The ForceSMIP project will ultimately produce estimates of the forced climate response and a repository of project contributors’ code submissions.
ForceSMIP, conceptualized by Robert Jnglin Wills of ETH Zürich Institute for Atmospheric and Climate Science and Clara Deser of the National Center for Atmospheric Research (NCAR), was jump-started in late August, 2023, via hackathon events at ETH and NCAR.
Several members of the Program on Climate Model Diagnosis and Intercomparison (PCMDI), which is funded by the Regional & Global Model Analysis (RGMA) program area, contributed to the Boulder hackathon event:
- Stephen Po-Chedley of Lawrence Livermore National Laboratory (LLNL) helped Deser and Wills organize the hackathon (along with Adam Phillips of NCAR, Karen McKinnon of the University of California, Los Angeles (UCLA), and Sebastian Sippel of the University of Leipzig);
- Elizabeth Barnes of Colorado State University (CSU) gave a lecture on ML methods; and
- Céline Bonfils of LLNL contributed to a “chalk talk” that covered detection and attribution methods.
PCMDI was established at LLNL in 1989 with the mission of developing advanced methods and tools to improve, diagnose, and enable the intercomparison of global climate models and earth system models. The goal is to advance climate models so that they are capable of addressing the critical questions that policymakers and society have about global and regional climate change.
During the ForceSMIP hackathon at the NCAR node, approximately 40 participants worked in small groups to develop methods to estimate the forced climate response. To help get participants started, organizers created example Jupyter Notebooks that applied current methods to address disentanglement problems, such as low frequency component analysis, regression techniques, artificial neural networks, and linear inverse models. Although these methods showed excellent skill in isolating the forced component of regional temperature change, there was room for improvement in estimating the forced pattern of tropical precipitation change.
PCMDI led and contributed to several hackathon groups that made excellent progress, creating novel methods to tackle the ForceSMIP hackathon challenge:
- Bonfils and Gavin Madakumbura of UCLA extended traditional detection and attribution techniques (e.g., Bonfils et al. 2020) and developed a novel implementation of an artificial neural network (Madakumbura et al. 2021); and
- Barnes’ group, including PCMDI postdoctoral researcher Martin Fernandez of CSU, worked collaboratively to develop ML algorithms to contribute to the ForceSMIP project.
The techniques collectively showed promise in isolating the forced component of tropical precipitation change, which was a challenge for existing methodologies.
Given the rapid evolution of ML, AI, and statistical learning, applying these tools to vast quantities of model simulation data may help shed light on important climate questions:
- To what degree is Atlantic decadal variability driven by aerosol forcing versus multidecadal variability?
- Does external forcing contribute to satellite-era cooling in the eastern tropical Pacific?
- How much does natural, internal variability contribute to known model-observational differences in the rate of climate change?
These climate puzzles affect our understanding of the efficacy of historical forcing, climate sensitivity, and the amplitude of internal variability. A better understanding of historical changes in climate will, therefore, improve our understanding of climate system properties and enhance future climate projections.